ROHM developed tens of milliwatts of ultra-low power consumption device side learning AI chip
Time:2022-10-03
Views:1696
ROHM has developed dozens of milliwatts of ultra-low power consumption device side learning AI chips, which can predict faults in real time on the device side without ECS
*On device learning: learning and training on the same AI chip
ROHM, a world-renowned semiconductor manufacturer (headquartered in Kyodo, Japan), has developed a device side learning * AI chip (SoC equipped with a device side learning AI accelerator). This product uses AI (artificial intelligence) technology to predict the faults (fault sign detection) of electronic devices such as built-in motors and sensors in real time with ultra-low power consumption, and is very suitable for edge computing devices and endpoints in the IoT field.
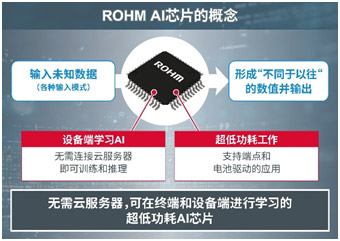
Generally, to achieve its functions, AI chips need to conduct "training" to set judgment criteria and "reasoning" to judge how to handle the information learned. In this case, "training" needs to gather a huge amount of data to form a database and update at any time. Therefore, the AI chip for training needs to have high computing power, and its power consumption will increase accordingly. Because of this, high-performance and expensive AI chips for cloud computing device development emerge in endlessly, while the development of low-power, device side learning AI chips for edge computing devices and endpoints (the key to building an Internet of Things society more effectively) is fraught with difficulties.
The AI chip developed this time is based on the "device side learning algorithm" developed by Professor Matsuma of Keio University in Japan, an AI accelerator * 2 (dedicated AI hardware computing circuit) for commercial development and a ROHM 8-bit efficient CPU "tinyMicon MatisseCORE" ™ (hereinafter referred to as "Matisse"). By combining 20000 ultra small AI accelerators with efficient CPUs, training and reasoning can be realized with ultra-low power consumption of only tens of mW (only 1/1000 of the previous AI training chips). With this product, unknown input data and patterns can be formed into "different from the past" values at the device terminal and output without connecting to the ECS, so real-time fault prediction can be realized in many applications.
In the future, ROHM plans to apply the AI accelerator of the AI chip to IC products to realize fault prediction of motors and sensors. It is planned to launch the product in 2023 and put it into mass production in 2024.
Professor Matsuma Hongji, Department of Information Engineering, Keio University of Japan, said: "With the development of 5G communication and digital twin * 3 and other Internet of Things technologies, the requirements for cloud computing are getting higher and higher. However, it is not realistic to process all data on the cloud server from the aspects of load, cost and power consumption. The ‘device side learning‘ we studied and the ‘device side learning algorithm‘ we developed are to improve the data processing efficiency at the edge and create a better Internet of Things society. This time, our school has connected with ROHM The research has further improved the device side learning circuit technology and is expected to launch products in a cost-effective manner. We expect that in the near future, this prototype AI chip will be successfully embedded in ROHM IC products, contributing to a more efficient Internet of Things society. "
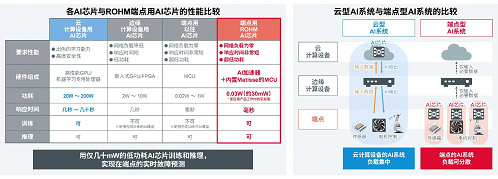
< About tinyMicon MatisseCORE ™>
tinyMicon MatisseCORE ™ (Matisse: Micro arithmetical unit for tiny size sequencer) is an 8-bit microprocessor (CPU) independently developed by ROHM, which aims to improve the intelligence of analog IC with the development of Internet of Things technology. With the instruction set optimized for embedded applications and the latest compiler technology, smaller chip area and program code, as well as higher speed operation processing capability are realized with high standards. In addition, the product also meets the requirements of the automotive functional safety standard "ISO 26262", ASIL-D, etc., and is suitable for applications requiring high reliability. In addition, with the built-in "real-time debugging function", the processing during debugging can not affect the operation of the application program at all, so debugging can be performed while the application product is working.
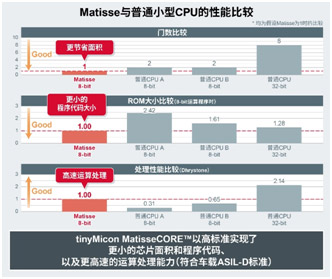
< Detailed introduction of AI chip (SoC equipped with device side learning AI accelerator) >
The prototype AI chip for device side learning (product model: BD15035) developed this time adopts the "device side learning algorithm (AI circuit of three-layer neural network * 4)" developed by Professor Matsuya of Keio University on the basis of artificial intelligence technology. In order to launch products that can be put on the market, ROHM reduced the size of this AI circuit from 5 million gates to 20000 gates, which is only 0.4% of the original size, and reconstructed it into its own AI accelerator "AxlCORE-ODL". At the same time, ROHM made use of its 8-bit efficient microprocessor "tinyMicon MatisseCORE" ™” The operation control of AI accelerator makes it possible to train and reason with ultra-low power consumption of only tens of milliwatts. With this product, unknown input data and modes (such as acceleration, current, illuminance, sound, etc.) can be formed into a value "different from the past (abnormal degree)" and output by the device terminal without connecting to the ECS and conducting AI training in advance. Therefore, not only can the ECS and communication costs be reduced, but also real-time fault prediction (fault sign detection) can be conducted through the terminal AI.
The prototype AI chip for device side learning (product model: BD15035) developed this time adopts the "device side learning algorithm (AI circuit of three-layer neural network * 4)" developed by Professor Matsuya of Keio University on the basis of artificial intelligence technology. In order to launch products that can be put on the market, ROHM reduced the size of this AI circuit from 5 million gates to 20000 gates, which is only 0.4% of the original size, and reconstructed it into its own AI accelerator "AxlCORE-ODL". At the same time, ROHM made use of its 8-bit efficient microprocessor "tinyMicon MatisseCORE" ™” The operation control of AI accelerator makes it possible to train and reason with ultra-low power consumption of only tens of milliwatts. With this product, unknown input data and modes (such as acceleration, current, illuminance, sound, etc.) can be formed into a value "different from the past (abnormal degree)" and output by the device terminal without connecting to the ECS and conducting AI training in advance. Therefore, not only can the ECS and communication costs be reduced, but also real-time fault prediction (fault sign detection) can be conducted through the terminal AI.
In addition, ROHM also provides an evaluation board that can install the expansion board for the microcontroller development board "Arduino * 5" (equipped with Arduino compatible pins), so that customers can evaluate this AI chip. The evaluation board is equipped with wireless communication modules (Wi Fi and Bluetooth ®) And 64kbit EEPROM (memory), just connect the evaluation board with the sensor and other units, install the sensor on the monitoring object, and then confirm the effect of AI chip on the display screen. For this evaluation board, please contact the sales department of ROHM if necessary.
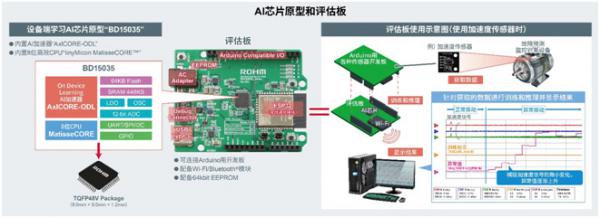
< Demo video of AI chip >
ROHM also prepared the demonstration video of the AI chip when using the evaluation board. Welcome to watch!
https://api01-platform.stream.co.jp/apiservice/plt3/NDc3%23MTY5MQ%3d%3d%23500%232d0%230%233FE6F851E400%23OzEwOzEwOzEw%23
< Explanation of terms >
*1) Edge computing devices and endpoints
Connect the servers and computers that constitute the big data foundation to the cloud, namely, "cloud servers" and "cloud computing devices". The edge computing devices that constitute the edge (end) side refer to the computers or devices of the terminal. Endpoints refer to devices and locations that are more extreme than edge computing devices.
*2) AI accelerator
When implementing the AI function, the software will change the processor (CPU) to a device (or electronic circuit) that improves the processing speed through hardware processing.
*3) Numerical twinning
*3) Numerical twinning
A technology that maps real world information into virtual space (digital space) like twins.
*4) Three layer neural network
In the neural network (mathematical formula and function model) inspired by human brain mechanism, in the processing flow composed of input layer, intermediate layer and output layer, the intermediate layer is regarded as a simple neural network consisting of one layer and only three layers in total. Multilayer neural networks with dozens of intermediate layers to perform more complex AI processing are called "deep learning".
*5) Arduino
Arduino‘s open source platform, which is composed of PCB board with microcontroller and I/O ports and software development environment, has been widely used in the world.
Disclaimer: This article is transferred from other platforms and does not represent the views and positions of this site. If there is any infringement or objection, please contact us to delete it. thank you! |